Evaluation of sparsity promoting algorithms for source location with spherical microphone array
DOI:
https://doi.org/10.55753/aev.v36e53.31Keywords:
sound source localization, sparsity regularization, spherical geometry of microphone arraysAbstract
When doing spatial audio processing of sound scenes it is often necessary to first detect the sound sources present in the scene, which is commonly done with the aid of a microphone array and a direction of arrival (DOA) estimation algorithm. If the system should be able to analyze sound coming from all possible directions, then spherical microphone arrays are most commonly used. Classical DOA algorithms, such as plane-wave decomposition or spherical beamforming, suffer from low localization accuracy. In an attempt to improve DOA estimation, the compressive beamforming (CB) algorithm has been proposed. CB applies sparsity regularization to regular beamforming through the use of L1-norm minimization, therefore taking into account the assumption that common sound scenes are usually composed of only a handful of sound sources.
In this paper, the performance of three sparsity regularization algorithms on a plane-wave decomposition model is compared: the L1-norm minimization via Disciplined Convex Program (DCP), the Least Absolute Shrinkage and Selection Operator (LASSO) method and the Orthogonal Matching Pursuit (OMP). It is shown that the three algorithms were able to estimate accurately the number of sources and its directions for an actificial sound scene, both with and without noise. However, the performance has deteriorated when applied at a practical situation with a sound source recorded in an anechoic chamber. In this case, there was an improvement with the combnation of the LASSO to estimate the number of sound sources and the OMP to refine the wave amplitude.
References
BENESTY, J.; CHEN, J.; HUANG, Y. Microphone Array Signal Processing. [S.l.]: Springer Berlin Heidelberg, 2008. (Springer Topics in Signal Processing). doi: 10.1007/978-3-540-78612-2. ISBN 978-3540786122. DOI: https://doi.org/10.1007/978-3-540-78612-2
NASCIMENTO, Vítor H.; MASIERO, Bruno S.; RIBEIRO, Flávio P. Acoustic imaging using the Kronecker array transform. In: COELHO, Rosangela Fernandes; NASCIMENTO, Vitor Heloiz; QUEIROZ, Ricardo Lopes de; ROMANO, Joao Marcos Travassos; CAVALCANTE, Charles Casimiro (Ed.). Signals and Images: Advances and Results in Speech, Estimation, Compression, Recognition, Filtering, and Processing. [S.l.]: CRC Press, 2015. p. 153–178. ISBN 978-1498722360. DOI: https://doi.org/10.1201/b19385-9
HÖGBOM, J. A. Aperture synthesis with a non-regular distribution of interferometer baselines. Astronomy and Astrophysics Supplement, v. 15, p. 417–426, 1974. ISSN 0365-0138. Disponível em: https://ui.adsabs.harvard.edu/abs/1974A%26AS...15..417H/.
WANG, Yanwei; LI, Jian; STOICA, Petre; SHEPLAK, Mark; NISHIDA, Toshikazu. Wide-band relax and wideband clean for aeroacoustic imaging. The Journal of the Acoustical Society of America, v. 115, n. 2, p. 757–767, 2004. doi: 10.1121/1.1639906. DOI: https://doi.org/10.1121/1.1639906
DOUGHERTY, Robert P. Extensions of DAMAS and benefits and limitations of deconvolution in beamforming. In: 11th AIAA/CEAS Aeroacoustics Conference (26th AIAA Aeroacoustics Conference). [S.l.: s.n.], 2005. p. 1–13. ISBN 156-3477300. ISSN 0146-3705. doi: 10.2514/6.2005-2961. DOI: https://doi.org/10.2514/6.2005-2961
YAN, Shefeng; MA, Yuanliang; HOU, Chaohuan. Optimal array pattern synthesis for broadband arrays. The Journal of the Acoustical Society of America, v. 122, n. 5, p. 2686–2696, 2007. ISSN 00014966. doi: 10.1121/1.2785037. DOI: https://doi.org/10.1121/1.2785037
EHRENFRIED, Klaus; KOOP, Lars. Comparison of iterative deconvolution algorithms for the mapping of acoustic sources. AIAA Journal, v. 45, n. 7, p. 1584–1595, 2007. ISSN 0001-1452. doi: 10.2514/1.26320. DOI: https://doi.org/10.2514/1.26320
YARDIBI, Tarik; LI, Jian; STOICA, Petre; CATTAFESTA, Louis N. Sparsity constrained deconvolution approaches for acoustic source mapping. The Journal of the Acoustical Society of America, v. 123, n. 5, p. 2631–2642, 2008. doi: 10.1121/1.2896754. DOI: https://doi.org/10.1121/1.2896754
XENAKI, Angeliki; GERSTOFT, Peter; MOSEGAARD, Klaus. Compressive beamforming. The Journal of the Acoustical Society of America, v. 136, n. 1, p. 260–271, 2014. ISSN 1520-8524. doi: 10.1121/1.4883360. DOI: https://doi.org/10.1121/1.4883360
Shi, J.; Hu, G.; Zhang, X.; Sun, F.; Zhou, H. Sparsity-based two-dimensional DOA estimation for coprime array: From sum–difference coarray viewpoint. IEEE Transactions on Signal Processing, v. 65, n. 21, p. 5591–5604, 2017. doi: 10.1109/TSP.2017.2739105. DOI: https://doi.org/10.1109/TSP.2017.2739105
EPAIN, Nicolas; JIN, Craig; SCHAIK, André van. The application of compressive sampling to the analysis and synthesis of spatial sound fields. In: 127th AES Convention. New York, USA: [s.n.], 2009. p. 1–12. Disponível em: http://www.aes.org/e-lib/browse.cfm?elib=15052.
FERNANDEZ-GRANDE, Efren; XENAKI, Angeliki. Compressive sensing with a spherical microphone array. The Journal of the Acoustical Society of America, v. 139, n. 2, p. EL45–EL49, 2016. doi: 10.1121/1.4942546.
PING, Guoli; CHU, Zhigang; YANG, Yang. Compressive spherical beamforming for acoustic source identification. Acta Acustica united with Acustica, v. 105, n. 6, p. 1000–1014, 2019. ISSN 1610-1928. doi:10.3813/AAA.919406. DOI: https://doi.org/10.3813/AAA.919406
PAN, J. Spherical harmonic atomic norm and its application to DOA estimation. IEEE Access, v. 7, p. 156555–156568, 2019. doi: 10.1109/ACCESS.2019.2950016. DOI: https://doi.org/10.1109/ACCESS.2019.2950016
CHEN, Scott Shaobing; DONOHO, David L.; SAUNDERS, Michael A. Atomic decomposition by basis pursuit. SIAM Review, v. 43, n. 1, p. 129–159, 2001. doi: 10.1137/S003614450037906X. DOI: https://doi.org/10.1137/S003614450037906X
GORODNITSKY, I. F.; RAO, B. D. Sparse signal reconstruction from limited data using FOCUSS: a re-weighted minimum norm algorithm. IEEE Transactions on Signal Processing, v. 45, n. 3, p. 600–616, 1997. doi: 10.1109/78.558475. DOI: https://doi.org/10.1109/78.558475
ERKOC, M. E.; KARABOGA, N. Evolutionary algorithms for sparse signal reconstruction. Signal, Image and Video Processing, p. 1863–1711, 2019. doi: 10.1007/s11760-019-01473-w. DOI: https://doi.org/10.1007/s11760-019-01473-w
ELAD, M. Sparse and Redundant Representations. [S.l.]: Springer-Verlag New York, 2010. (Springer Topics in Signal Processing). doi: 10.1007/978-1-4419-7011-4. ISBN 978-1441970114. DOI: https://doi.org/10.1007/978-1-4419-7011-4
FERNANDEZ-GRANDE, Efren; XENAKI, Angeliki. Compressive sensing with a spherical microphone array. The Journal of the Acoustical Society of America, v. 139, p. EL45–EL49, 02 2016. doi: 10.1121/1.4942546. DOI: https://doi.org/10.1121/1.4942546
GERSTOFT, Peter; XENAKI, Angeliki; MECKLENBRäUKER, Christoph. Multiple and single snapshot compressive beamforming. The Journal of the Acoustical Society of America, v. 138, n. 4, p. 2003–2014, 2015. doi: 10.1121/1.4929941. DOI: https://doi.org/10.1121/1.4929941
ROSSUM, Guido Van; DRAKE, Fred L. Python 3 Reference Manual. Scotts Valley, USA: CreateSpace, 2009. ISBN 978-1441412690.
TREES, Harry L. Van. Optimum Array Processing: Part IV of Detection, Estimation, and Modulation Theory. John Wiley & Sons, 2002. ISBN 9780470542965. Disponível em: https://books.google.com.br/books?id=J5TZDwAAQBAJ.
CANDÈS, Emmanuel J.; WAKIN, M. B. An Introduction To Compressive Sampling. IEEE Signal Processing Magazine, v. 25, n. 2, p. 21–30, 2008. ISSN 1053-5888. doi: 10.1109/MSP.2007.914731. Disponível em: http://ieeexplore.ieee.org/lpdocs/epic03/wrapper.htm?arnumber=4472240. DOI: https://doi.org/10.1109/MSP.2007.914731
RAFAELY, B. Fundamentals of Spherical Array Processing. [S.l.]: Springer International Publishing, 2018. (Springer Topics in Signal Processing). doi: 10.1007/978-3-662-45664-4. ISBN 978-3319995618. DOI: https://doi.org/10.1007/978-3-319-99561-8
SAFF, E.B.; KUIJLAARS, A.B.J. Distributing many points on a sphere. The Mathematical Intelligencer, v. 19, p. 5–11, 1997. doi: 10.1007/BF03024331. DOI: https://doi.org/10.1007/BF03024331
RAFAELY, Boaz. Fundamentals of Spherical Array Processing. Berlin, Heidelberg: Springer Berlin Heidelberg, 2015. v. 8. (Springer Topics in Signal Processing, v. 8). doi: 10.1007/978-3-662-45664-4. ISSN 09250042. ISBN 978-3662456637. DOI: https://doi.org/10.1007/978-3-662-45664-4
BERTET, Stéphanie; DANIEL, Jerome; PARIZET, Etienne; WARUSFEL, Olivier. Investigation on localisation accuracy for first and higher order ambisonics reproduced sound sources. Acta Acustica united with Acustica, v. 99, n. 4, p. 642 – 657, 2013. doi: 10.3813/AAA.918643. DOI: https://doi.org/10.3813/AAA.918643
ELAD, M. Optimized projections for compressed sensing. IEEE Transactions on Signal Processing, v. 55, n. 12, p. 5695–5702, 2007. doi: 10.1109/TSP.2007.900760. DOI: https://doi.org/10.1109/TSP.2007.900760
DIAMOND, Steven; BOYD, Stephen. CVXPY: A Python-embedded modeling language for convex optimization. Journal of Machine Learning Research, v. 17, n. 1, p. 2909–2913, 2016. ISSN 1532-4435. Disponível em: https://dl.acm.org/doi/10.5555/2946645.3007036.
TROPP, J. A.; GILBERT, A. C. Signal recovery from random measurements via orthogonal matching pursuit. IEEE Transactions on Information Theory, v. 53, n. 12, p. 4655–4666, 2007. doi: 10.1109/TSP.2015.2413384. DOI: https://doi.org/10.1109/TIT.2007.909108
PEDREGOSA, Fabian; VAROQUAUX, Gaël; GRAMFORT, Alexandre; MICHEL, Vincent; THIRION, Bertrand; GRISEL, Olivier; BLONDEL, Mathieu; PRETTENHOFER, Peter; WEISS, Ron; DUBOURG, Vincent; VANDERPLAS, Jake; PASSOS, Alexandre; COURNAPEAU, David; BRUCHER, Matthieu; PERROT, Matthieu; DUCHESNAY Édouard. Scikitlearn: Machine learning in Python. Journal of Machine Learning Research, v. 12, n. oct, p. 2825–2830, 2011. Disponível em: http://jmlr.org/papers/v12/pedregosa11a.html.
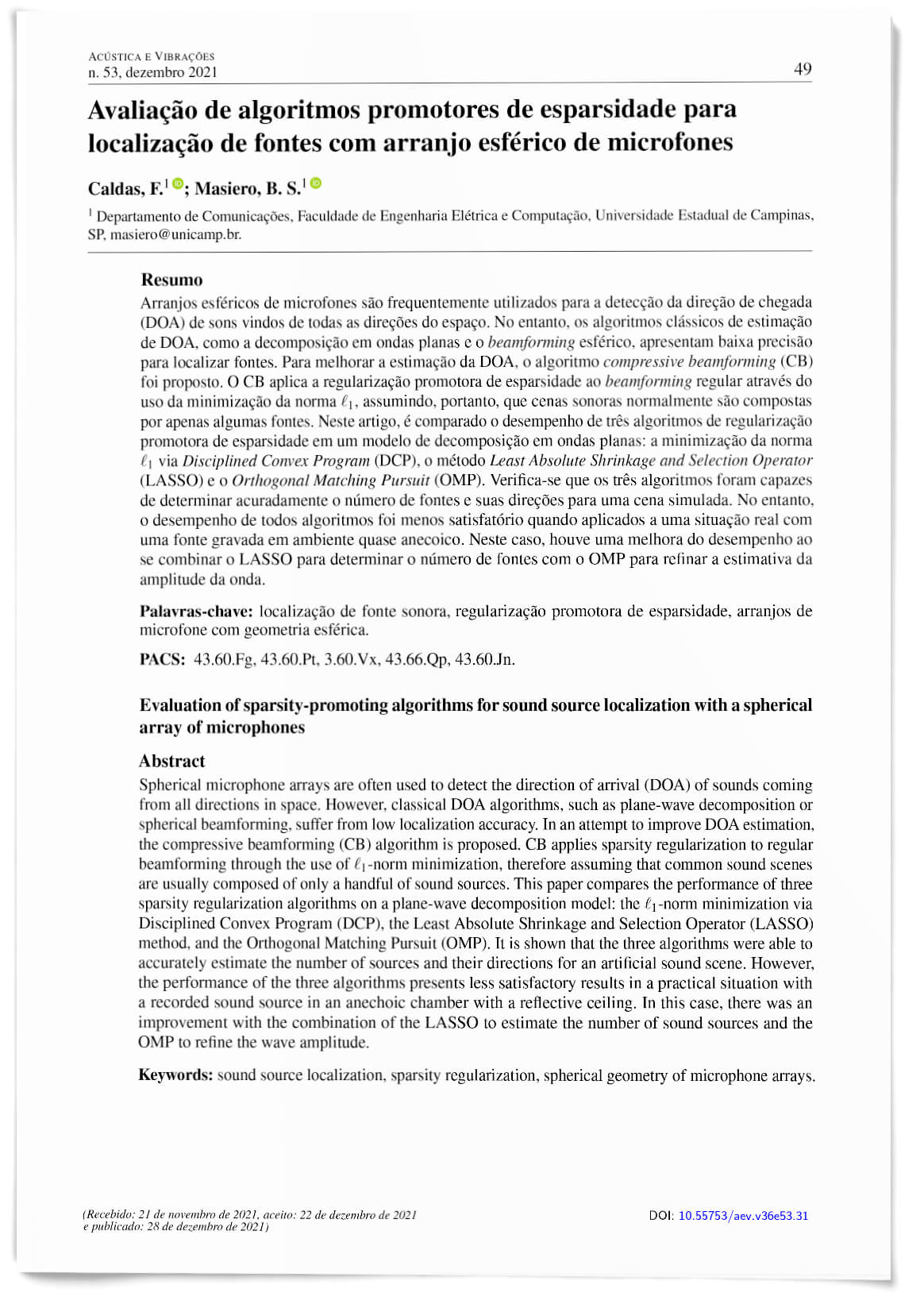
Downloads
Published
How to Cite
Issue
Section
License
Copyright (c) 2021 Acústica e Vibrações

This work is licensed under a Creative Commons Attribution-NonCommercial-ShareAlike 4.0 International License.