The effects of anger on automated long-term-spectra based speaker-identification
DOI:
https://doi.org/10.55753/aev.v37e54.193Keywords:
automated speaker identification, long term spectra, forensic acoustics, emotional distortions, angerAbstract
Forensic speaker identification has traditionally considered approaches based on long-term (a few tens of seconds) spectra analysis as especially robust. This is because they work well for short recordings, are not sensitive to changes in the intensity of the sample, and continue to function in the presence of noise and limited passband. Because of this, the long-term spectra approach is one of the preferred tools for forensic speaker identification, in addition to formant analysis, speed of speech, and determination of the fundamental frequency. However, we find that anger induces a significant distortion of the acoustic signal for long-term spectra analysis purposes. Even moderate anger offsets speaker identification results by 33% in the direction of a different speaker altogether (in the space of sample correlations). Therefore, caution should be exercised when applying this tool.
References
HOLLIEN, Harry. Barriers to Progress in Speaker Identification with Comments on the Trayvon Martin Case. Linguistic Evidence in Security, Law and Intelligence, University Library System, University of Pittsburgh, v. 1, n. 1, p. 76–98, dez. 2013. ISSN 2327-5596. doi: 10.5195/lesli.2013.3. DOI: https://doi.org/10.5195/LESLI.2013.3
HOLLIEN, Harry. An Approach to Speaker Identification. Journal of Forensic Sciences, Wiley, v. 61, n. 2, p. 334–344, fev. 2016. doi: 10.1111/1556-4029.13034, pMID: 27404606. DOI: https://doi.org/10.1111/1556-4029.13034
HOLLIEN, Harry Francis. Forensic Voice Identification. Londres, Inglaterra: Academic Press, 2002. ISBN 0123526213.
WILLIAMS, Carl E.; STEVENS, Kenneth N. Emotions and speech: Some acoustical correlates. The Journal of the Acoustical Society of America, Acoustical Society of America (ASA), v. 52, n. 4B, p. 1238–1250, out. 1972. doi: 10.1121/1.1913238. DOI: https://doi.org/10.1121/1.1913238
BANSE, Rainer; SCHERER, Klaus R. Acoustic profiles in vocal emotion expression. Journal of Personality and Social Psychology, American Psychological Association (APA), v. 70, n. 3, p. 614–636, 1996. doi: 10.1037/0022- 3514.70.3.614. DOI: https://doi.org/10.1037//0022-3514.70.3.614
n.◦ 54, dezembro 2022 DOI: https://doi.org/10.54580/R0402
JOHNSTONE, Tom. The effect of emotion on voice production and speech acoustics. Tese (PhD) — University of Western Australia & University of Geneva, Perth, Australia, 2001. doi: https://doi.org/10.31237/osf.io/qd6hz. DOI: https://doi.org/10.31237/osf.io/qd6hz
SCHERER, Klaus R. Voice, Stress, and Emotion. In: . Dynamics of Stress: Physiological, Psychological and Social Perspectives. 1. ed. [S.l.]: Springer US, 1986. p. 157–179. ISBN 978-1-4684-5122-1. doi: 10.1007/978-1-4684- 5122-1_9.
MARTIN, Maryanne. On the induction of mood. Clinical Psychology Review, Elsevier BV, v. 10, n. 6, p. 669–697, jan. 1990. ISSN 1873-7811. doi: 10.1016/0272-7358(90)90075-l. DOI: https://doi.org/10.1016/0272-7358(90)90075-L
HOLLIEN, Harry; MAJEWSKI, Wojciech. Speaker identification by long-term spectra under normal and distorted speech conditions. The Journal of the Acoustical Society of America, Acoustical Society of America (ASA), v. 62, n. 4, p. 975–980, out. 1977. ISSN 1520-8524. doi: 10.1121/1.381592. DOI: https://doi.org/10.1121/1.381592
KINNUNEN, Tomi; HAUTAMAKI, Ville; FRANTI, Pasi. On the Use of Long-Term Average Spectrum in Automatic Speaker Recognition. In: Proc. International Symposium on Chinese Spoken Language Processing. [s.n.], 2006. p. 559–567. Disponível em: https://citeseerx.ist.psu.edu/document?repid=rep1&type=pdf&doi=d3b4740466aeb1d25831b6329599b615a5bab9b1.
ORTEGA-RODRIGUEZ, Manuel. Relatório final: Articulação de um sistema de identificação de locutor para fins forenses (Original: Informe Final: Articulación de un sistema de identificación de locutor con fines forenses). [S.l.], 2016. Acessado em novembro de 2021. Disponível em: https://hdl.handle.net/10669/85190.
HARMEGNIES, Bernard. SDDD: A new dissimilarity index for the comparison of speech spectra. Pattern Recognition Letters, Elsevier BV, v. 8, n. 3, p. 153–158, out. 1988. ISSN 1872-7344. doi: 10.1016/0167-8655(88)90093-1. DOI: https://doi.org/10.1016/0167-8655(88)90093-1
STANTON, Jeffrey M. Galton, Pearson, and the Peas: A Brief History of Linear Regression for Statistics Instructors. Journal of Statistics Education, Informa UK Limited, v. 9, n. 3, jan. 2001. ISSN 1069-1898. doi: 10.1080/10691898.2001.11910537. DOI: https://doi.org/10.1080/10691898.2001.11910537
FULLER, Fred H. Detection of emotional stress by voice analysis final report. Bethesda, Maryland, USA, 1972. Disponível em: https://www.ojp.gov/ncjrs/virtual-library/abstracts/detection-emotional-stress-voice-analysis-final-report.
HARNSBERGER, James D.; HOLLIEN, Harry; MARTIN, Camilo A.; HOLLIEN, Kevin A. Stress and Deception in Speech: Evaluating Layered Voice Analysis. Journal of Forensic Sciences, Wiley, v. 54, n. 3, p. 642–650, maio 2009. ISSN 1556-4029. doi: 10.1111/j.1556-4029.2009.01026.x. DOI: https://doi.org/10.1111/j.1556-4029.2009.01026.x
PITTAM, Jeffery. The Long-Term Spectral Measurement of Voice Quality as a Social and Personality Marker: A Review. Language and Speech, SAGE Publications, v. 30, n. 1, p. 1–12, jan. 1987. ISSN 1756-6053. doi: 10.1177/002383098703000101. DOI: https://doi.org/10.1177/002383098703000101
RODMAN, Robert D.; POWELL, Michael S. Computer Recognition of Speakers Who Disguise Their Voice. In: The International Conference on Signal Processing Applications and Technology (ICSPAT 2000). [s.n.], 2000. Disponível em: https://api.semanticscholar.org/CorpusID:16980245.
HERTRICH, I.; ZIEGELMAYER, G. Sexual dimorphism in the long term speech spectrum. Human Evolution, Springer Science and Business Media LLC, v. 2, n. 3, p. 255–262, maio 1987. doi: 10.1007/bf03016110. DOI: https://doi.org/10.1007/BF03016110
LINVILLE, Sue Ellen. Source Characteristics of Aged Voice Assessed from Long-Term Average Spectra. Journal of Voice, Elsevier BV, v. 16, n. 4, p. 472–479, dez. 2002. doi: 10.1016/s0892-1997(02)00122-4. DOI: https://doi.org/10.1016/S0892-1997(02)00122-4
YÜKSEL, Mustafa; GÜNDÜZ, Bülent. Long term average speech spectra of Turkish. Logopedics Phoniatrics Vocology, Informa UK Limited, v. 43, n. 3, p. 101–105, set. 2017. doi: 10.1080/14015439.2017.1377286. DOI: https://doi.org/10.1080/14015439.2017.1377286
National Institute of Standards and Technology. NIST/SEMATECH e-Handbook of Statistical Methods. [s.n.], 2012. Acessado em outubro de 2021. Disponível em: https://www.itl.nist.gov/div898/handbook/prc/section2/prc222.htm.
Audacity Team. Audacity (v. 2.1.0), editor e gravador de áudio. 2015. Disponível em: https://www.audacityteam.org/.
The International Association for Forensic Phonetics and Acoustics. Code of Practice. [S.l.], 2004. Acessado em janeiro de 2018. Disponível em: https://www.iafpa.net/the-association/code-of-practice/.
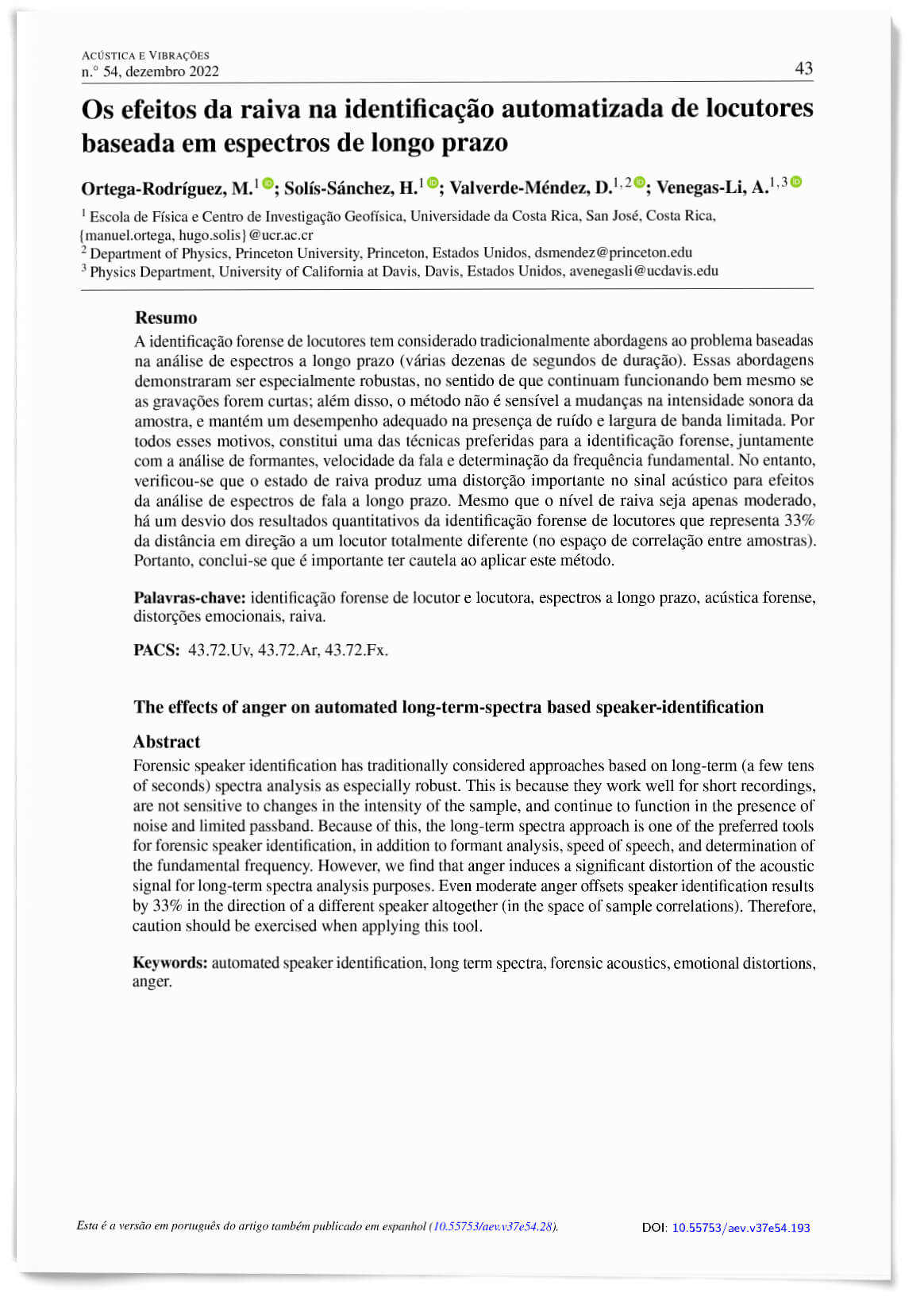
Published
How to Cite
Issue
Section
License
Copyright (c) 2022 Acoustics and Vibrations (Acústica e Vibrações)

This work is licensed under a Creative Commons Attribution-NonCommercial-ShareAlike 4.0 International License.