Fast technique for auralization generation using artificial neural networks
DOI:
https://doi.org/10.55753/aev.v33e50.84Keywords:
acoustic virtual reality, room auralization, BRIRs generation, artificial neural networks, room acoustic simulation, articulation indexAbstract
The main goal in development of numerical techniques in acoustic virtual reality systems and production of reliable auralizations is to reduce the computational cost and, at the same time, to guarantee the sound quality. In this paper, a new technique for modeling head-related transfer functions are presented. Artificial neural networks of the radial basis functions type are used. A set of these networks is trained and tested to cover the entire auditory space around the head. Each neural network for a given direction has as input the spectrum of the sound ray that reaches the receiver and, as output, the filtered head-related impulse response, for the corresponding direction working directly in the time domain and circumventing the need for convolutions with a computational cost reduction of 90%. The proposed technique is compared with the convolution method in both the time and frequency domain. The results show the efficiency of the proposed technique, with correlation values very close to one. To validate the result, preliminary tests using articulation indices to compare speech intelligibility in an actual and virtual room were conducted, with fully satisfactory results.
References
VORLÄNDER, M. Auralization: Fundamentals of Acoustics, Modelling, Simulation, Algorithms and Acoustic Virtual Reality. Berlin: Springer-Verlag, 2008. doi: 10.1121/1.2908264 DOI: https://doi.org/10.1121/1.2908264
KLEINER, M.; DALENBÄCK, B.I.; SVESSON, P. Auralization - an overview. J. audio Eng. Soc, 41, p.861, 1993.
BLAUERT, J. Spatial Hearing. Cambridge: The MIT Press, 1997. doi: 10.7551/mitpress/6391.001.0001 DOI: https://doi.org/10.7551/mitpress/6391.001.0001
RINDEL, J. The use computer modeling in room acoustics. Journal of Vibro engeneering, 4(3):41–72, 2000.
TENENBAUM, R.A.; CAMILO, T.S.; TORRES, J.C.B. and GERGES, S.Y. Hybrid method for numerical simulation of room acoustic: Part 1 – theorical and numerical aspects. J. Braz. Soc. Mech. Sci. Engin., 29(2):211–221, 2007a. DOI: https://doi.org/10.1590/S1678-58782007000200012
BORK, I. Report on the 3rd Round Robin on room acoustical computer simulation - Part II: Calculations. Acta Acustica united with Acustica, 91(4):753–763, 2005.
SAVIOJA, L.; SVENSSON, U.P. Overview of geometrical room acoustic modeling techniques. J. Acoust. Soc. Am, 138(2):708–730, 2015 doi: 10.1121/1.4926438 DOI: https://doi.org/10.1121/1.4926438
EMBRECHTS, J. Randomly traced sound ray techniques. Acustica, 51:285–295, 1982.
KULOWSKI, A. Algorithmic representation of the ray tracing technique. Applied Acoustics, 18:449–469, 1984. doi: 10.1016/0003-682X(85)90024-6 DOI: https://doi.org/10.1016/0003-682X(85)90024-6
ONDET, M; BARBRY, J.L. Modeling of sound propagation in fitted workshops using ray tracing. J. Acoust. Soc. Am., 85(2):787–796, 1989. doi: 10.1121/1.397551 DOI: https://doi.org/10.1121/1.397551
FARINA, A. RAMSETE – a new pyramid tracer for medium and large-scale acoustic problems. Proceedings of the Euronoise, Lyon, 1995
ALLEN, J.B.; BERKLEY, D.A. Image method for efficiently simulating small-room acoustics. J. Acoust. Soc. Am., 65, p. 943, 1979. doi: 10.1121/1.382599 DOI: https://doi.org/10.1121/1.382599
DALENBÄCK, B; KLEINER, M.; SVENSON, P. A macroscopic view of diffuse reflection. J. áudio Eng. Soc. 42:793–807, 1994.
KURZINS, E.; FRICKE, F. The prediction of sound fields in non-diffuse spaces by random walk approach. J. Sound and Vib., 81(4):549–564, 1982. doi: 10.1016/0022-460X(82)90296-6 DOI: https://doi.org/10.1016/0022-460X(82)90296-6
ALARCÃO, D.; BENTO COELHO, J.L.; TENENBAUM, R.A. On modeling of room acoustics by a sound energy transition approach. Proceedings of EEA Symposium on Architectural Acoustics, 2000.
GARDNER, B.; MARTIN, K. HRTF Measurements of a KEMAR Dummy-Head Microphone. J. Acoust. Soc. Am., vol. 97, n. 6, pp. 3907– 3908, 1995. DOI: https://doi.org/10.1121/1.412407
BRINKMANN, F.; LINDAU, A.; WEINZIERL, S.; VAN DER PAR, S.; OPDAM, R.; VORLÄNDER, M. The FABIAN head-related transfer function database. doi: 10.14279/depositonce-5718.2, 2017.
MILLS, A. On the minimum audible angle. J. Acoust. Soc. Am., 30:237–246, 1958. doi: 10.1121/1.1909553 DOI: https://doi.org/10.1121/1.1909553
KISTLER, D. J.; WIGHTMAN, F.L. A model of head-related transfer functions based on principal components analysis and minimum-phase reconstruction. J. Acoust. Soc. Am. 91(3), 1637–1647, 1992. doi: 10.1121/1.402444 DOI: https://doi.org/10.1121/1.402444
TORRES, J.C.B; PETRAGLIA, M.R.; TENENBAUM, R.A. An efficient wavelet based HRTF model for auralization. Acta Acustica united with Acustica, 90(1):108−120, 2004.
HU, H.; ZHOU, L.; MA, H.; WU, Z. HRTF personalization based on artificial neural network in individual virtual auditory space. Applied Acoustics, 69(2):163–172, 2008. doi: 10.1016/j.apacoust.2007.05.007 DOI: https://doi.org/10.1016/j.apacoust.2007.05.007
TENENBAUM, R.A.; TAMINATO, F.O.; MELO,V.S.G.; TORRES, J.C.B. Auralization generated by modeling HRIRs with artificial neural networks and its validation using articulation tests. Applied Acoustics, 130, pp. 260–269, 2018. doi: 10.1016/j.apacoust.2017.09.025 DOI: https://doi.org/10.1016/j.apacoust.2017.09.025
BROOMHEAD, D.; LOWE, D. Multivariable functional interpolation and adaptive networks. Complex Systems, 2:321–355, 1988.
LI, L.; HUANG, Q. HRTF personalization modeling based on RBF neural network. Proceedings of IEEE International Conference on Acoustics, Speech and Signal Processing, p. 3707–3710, Vancouver, Canada, 2013. doi: 10.1109/ICASSP.2013.6638350 DOI: https://doi.org/10.1109/ICASSP.2013.6638350
TENENBAUM, R.A.; CAMILO, T.S.; TORRES, J.C.B. and STUTZ, L.T. Hybrid method for numerical simulation of room acoustic: Part 2 – validation of the computational code RAIOS 3. J. Braz. Soc. Mech. Sci. Engin., 29(2):223–231, 2007b. doi: 10.1590/S1678-58782007000200013 DOI: https://doi.org/10.1590/S1678-58782007000200013
FERNANDES, K.M.; TENENBAUM, R.A.; STUTZ, L.T. Problema inverso em propagação de ondas em sólidos com aplicação de método híbrido de otimização. Em: Técnicas de Inteligência Computacional com Aplicações em Problemas Inversos de Engenharia. Editora Omnipax, Curitiba, pp. 51–66, 2014. DOI: https://doi.org/10.7436/2014.tica.05
RAHIM, M.G., GOODYEAR, C.C. and KLEIJN, W.B. On the use of neural networks in articulatory speech synthesis. J. Acoust. Soc. Am., v. 93(2):1109–1121, 1992. DOI: https://doi.org/10.1121/1.405559
HAYKIN, S. Neural Networks and Learning Machines. 3rd Edition, Prentice Hall, New Jersey, 2009.
TAMINATO, F.O. Redes neurais artificiais aplicadas à modelagem de respostas impulsivas associadas à cabeça humana para gerar aurilização. Tese de doutorado, Programa de Pós-graduação em Modelagem Computacional, Universidade do Estado do Rio de Janeiro, 2018.
TENENBAUM, R.A.; TAMINATO, F.O.; MELO V.S.G. Fast auralization using radial basis functions type of artificial neural network techniques. Applied Acoustics, 157, 106993, 2020. doi: 10.1016/j.apacoust.2019.07.041 DOI: https://doi.org/10.1016/j.apacoust.2019.07.041
A Ground Truth for Room Acoustic Simulation. Outubro 2019. doi.org/10.14279/depositonce-6726
HODGSON, M.; YORK, N.; YANG, W.; BLISS, M. Comparison of predicted, measured, and auralized sound fields with respect to speech intelligibility in classrooms using CATT-acoustic and ODEON. Acta Acustica united with Acustica, 94(6):883–890, 2008. doi: 10.3813/AAA.918106 DOI: https://doi.org/10.3813/AAA.918106
MELO, V. S. G.; TENENBAUM, R. A.; NARANJO, J. F. L. A new approach to validate computer modeling auralizations by using articulation indexes. Acústica & Vibrações, Vol. 46, pp. 1−8, 2014. doi: 10.55753/aev.v29e46.125
POLLOW, M.; BEHLER, G. Variable directivity for platonic sound sources based on spherical harmonics optimization. Acta Acustica united with Acustica, 95(6):1082–1092, 2009. doi: 10.3813/AAA.918240 DOI: https://doi.org/10.3813/AAA.918240
KLEIN, J.; POLLOW, M.; VORLÄNDER, M. Optimized spherical sound source for auralization with arbitrary source directivity. Proceedings of the EAA Joint Symposium on Auralization and Ambisonics, p.56–61, Berlin, 2014. doi: 10.14279/depositonce-10
LINDAU, A.; ERBES, V.; LEPA, S.; MAEMPEL, H.J.; BRINKMANN, F.; WEINZIERL, S. A spatial audio quality inventory for virtual acoustic environments (SAQI). Acta Acustica united with Acustica, 100(5):984–994, 2014. doi: 10.3813/AAA.918778 DOI: https://doi.org/10.3813/AAA.918778
MELO, V. S. G.; LIMA, P. G.; SANTOS, T. C.; TENENBAUM, R. A. Validação de realidade virtual acústica via testes de articulação em salas ruidosas e reverberantes. Acústica & Vibrações, 49, pp. 51–57, 2017. doi: 10.55753/aev.v32e49.95 DOI: https://doi.org/10.55753/aev.v32e49.95
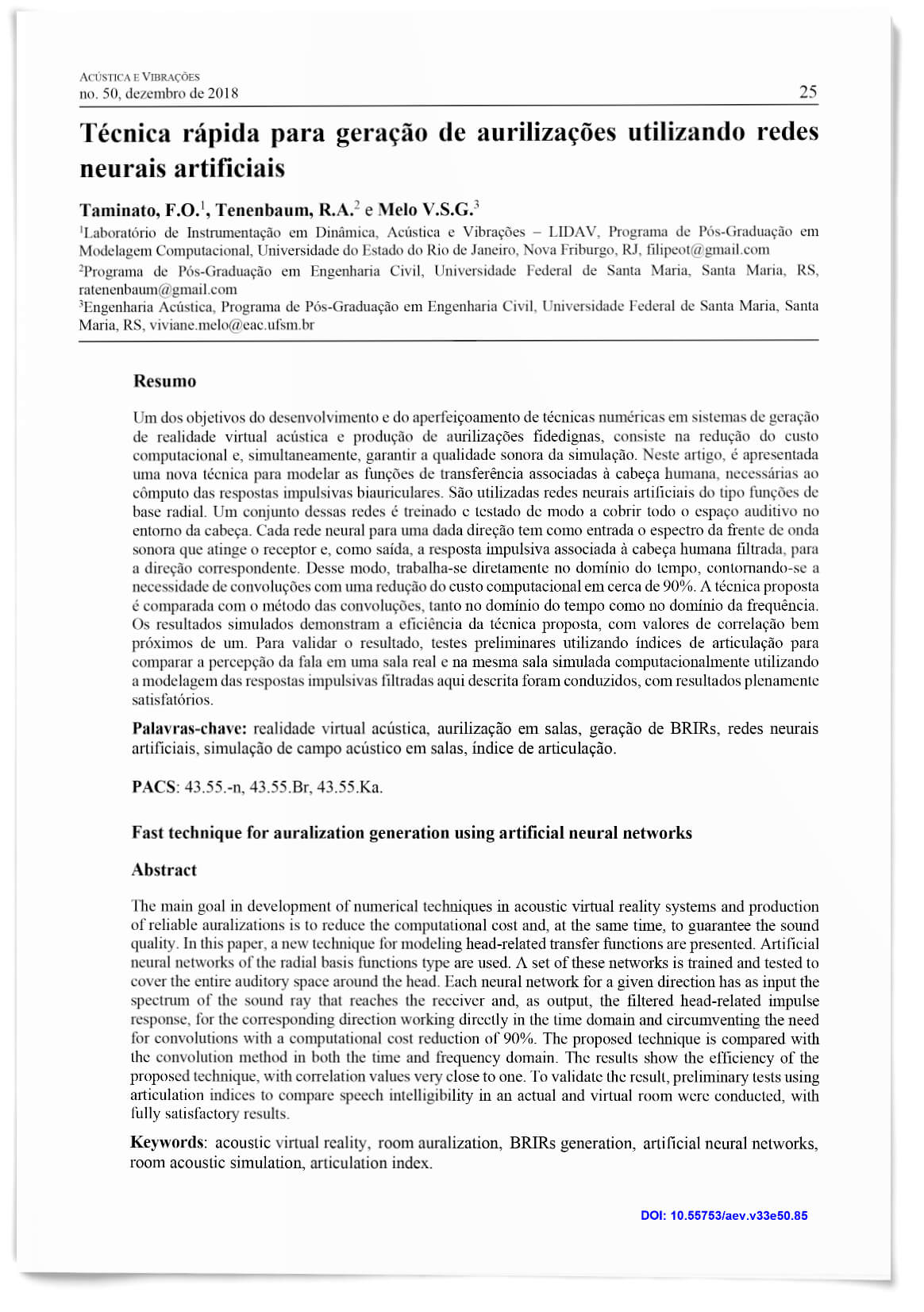
Downloads
Published
How to Cite
Issue
Section
License
Copyright (c) 2018 Acústica e Vibrações

This work is licensed under a Creative Commons Attribution-NonCommercial-ShareAlike 4.0 International License.